Main Content
Frequently Asked Questions (FAQ)
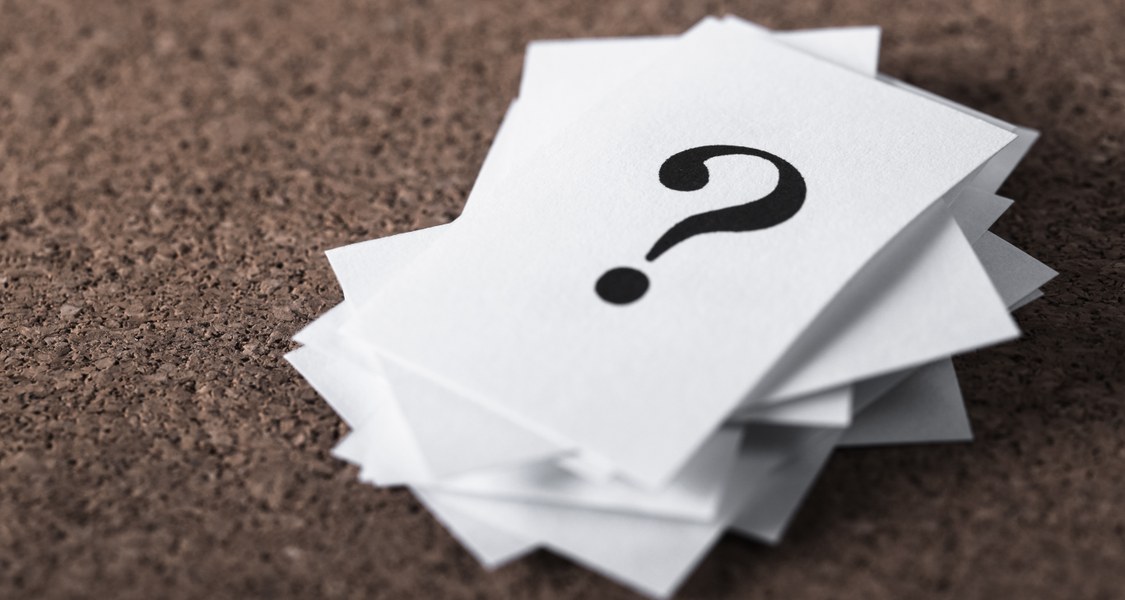
Here you can find answers to the most important questions about research data management.
Introduction and Background
What is research data?
What is research data management?
Why is research data management important for me?
What do I need to consider when planning?
How do I create a data management plan?
What are the requirements of research funding, publishers and the university?
Storing and Archiving Research Data
How do I structure my data in a useful way?
Which file formats should be used?
Where do I save my data during the work process?
What should I consider when backing up my data?
Where do I archive my data in the long term?
Publishing and Sharing Research Data
Why should I publish my data?
Does anything speak against publication?
What data protection restrictions must I have observe?
Who may decide on sharing and publishing of data?
Do I own the copyright to my data?
Can I control the use of my data?
Which licence should I choose?
How can I publish data?
How do I find a suitable repository?
What must I consider when feeding data into a repository?
What are metadata, metadata schemas, controlled vocabularies and documentation?
What are persistent identifiers?
Finding and Using Research Data
Where do I find research data?
How do I cite research data?
Introduction and Background
What are research data?
Research data are (digital) data that are created during a research process or are a result thereof. Since a wide variety of methods such as measurements, source research or surveys are used to obtain them, research data are always subject and project-specific. Further information on the definition of research data can be found below.
What is research data management?
Research data management aims at the responsible, planned and sustainable handling of these data, taking into account the entire data lifecycle (Fig. 1).
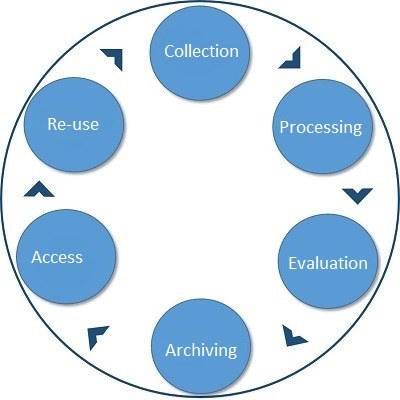
Fig. 1: Research data life cycle
Research data management therefore ideally begins with the planning of data collection and not only deals with technical storage and archiving, but also directs attention to making the data findable, accessible, comprehensible in terms of content and thus usable in the long term. Further information on research data management can be found here.
Why is research data management important for me?
The following reasons speak for systematic research data management and at the same time underline its importance for good scientific practice:
Research funding: Research data management, in some cases also data sharing, is often required by research funding bodies in order to enable the validation of results and to avoid multiple funding. (What are the requirements of funding bodies, publishers and universities?)
Re-usability: Good research data management minimises the risk of data loss and ensures the long-term usability of data, the acquisition of which is usually complex and associated with high costs, over 10 years and beyond, as required by good scientific practice.
Reproducibility: If experimentally obtained research data are maintained appropriately, a long-term reproducibility of results is possible.
Verifiability: The documentation of research data and its creation also makes results verifiable in the long term.
Citability: Data publications are fully citable as independent publications and thus increase the visibility of one's own research.
The following figure also shows the goals that can be pursued with research data management in different contexts:
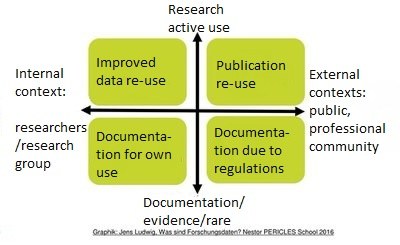
Fig. 2: Goals that can be pursued with RDM
What do I have to consider when planning?
Ideally, research data management should not only start after the data has been collected. Rather, considerations should be made about how to deal with the data even before it is collected. The data life cycle can serve as orientation.
Ideally, research data management should not only start after the data has been collected. Rather, considerations should be made about how to deal with the data even before it is collected. The data life cycle can serve as orientation.
The following guide from the Universität Kassel provides an overview of what should be considered when conducting research data management:
-
Determine who is responsible for setting up and controlling research data management at your institution.
-
Check whether there are specific institutional or general guidelines or proposals on research data management for your research field.
-
For each research project, establish as early as possible what obligations you are subject to, regarding the storage and publication of research data. (What are the requirements of funding bodies, publishers and the university?)
-
Identify what research data will be collected in the research project.
-
Consider which of the research data should be published and made available to others for re-use.
-
Think about how you want to store and archive research data. (Storing and archiving research data)
-
Check which storage and archiving options are available to you. Could you use a general or subject-specific data repository? (How do I find a suitable repository?)
-
Clarify the legal issues regarding the storage and dissemination of research data. This may concern data protection and copyright law, among other things
-
Create a data management plan. This documents your decisions and serves as proof of implementation. (How do I create a data management plan?)
Update the data management plan as the research progresses.
How do I create a data management plan?
A data management plan documents the handling of research data from the planning to the completion of a research project. However, it is a “living document” that can and must be adapted to changes, new findings or problems.
The following tools will help you to create data management plans:
- RDMO
The Research Data Management Organiser (RDMO) was developed by the Leibniz Institute for Astrophysics in Potsdam and the Potsdam University of Applied Sciences. The DFG-funded project is currently in its second phase. RDMO not only enables the creation of a data management plan in accordance with the requirements of various research funding programmes, but also supports researchers in the planning, implementation and administration of all research data management tasks. -
DMPonline
DMPonline is run by the UK Digital Curation Centre (DCC). The application is primarily tailored to the situation in the UK but can also be used for Horizon 2020 projects. For German-speaking countries, the Humboldt University of Berlin provides a handout on how to use DMPonline for Horizon 2020 (PDF). -
DMPTool
DMPTool is operated by the California Digital Library. The website also offers examples of data management plans. The tool is oriented towards the funding landscape in the USA and is therefore of limited use for German or European projects. -
ARGOS
ARGOS is a European online tool developed by OpenAIRE to create data management plans, based on the open-source software OpenDMP. The registration is designed to be open, as you can log in directly with your ORCiD ID, Google account or even Facebook or Twitter account. Data management plans created with ARGOS are also internationally connectable, as they are directly available in the DMP metadata standard of the Research Data Alliance (RDA). -
The following checklists, models, templates and wizards provide further assistance in creating data management plans:
Checklists:
- Checklist of the Digital Curation Centre of the University of Edinburgh (PDF)
- Questionnaire “Twenty Questions for Research Data Management” (PDF) by Oxford zoologist David Shotton
-
Patterns & Templates:
- DMP Catalogue of the LIBER Research Data Management Working Group (PDF)
- Sample DMP for a BMBF application (PDF)
- Sample DMP for a DFG proposal (PDF)
- Model DMP for Horizon 2020 Version 3.0 (PDF)
- Search on Zenodo.org with the keyword “DMP”
- Horizon 2020 template (PDF)
- Presentation of a data management plan for RWTH Aachen University (PDF)
-
Wizards:
- The following examples of data management plans are also helpful:
-
The short video tutorial from HU-Berlin also provides a good introduction to the topic of “data management plans.”
What are the requirements of research funding, publishers and the university?
- German Research Foundation (DFG)
In its 'Guidelines for Safeguarding Good Scientific Practice', which came into force on 1 August 2019, the DFG makes fundamental stipulations on the handling of research data and requires, among other things, that “research data [...] are generally kept accessible and traceable [...] for a period of ten years”.
Furthermore, the guideline states:
“For reasons of traceability, connectivity of research and re-usability, researchers deposit the research data on which the publication is based [...] accessibly in recognised archives and repositories whenever possible.”
The 'Guidelines for Handling Research Data' (PDF) adopted in 2015 contain further recommendations for the provision of data, but also for data-related project planning. Among other things, they state:
“Already in the planning phase of a project, consideration should be given to whether and which of the research data resulting from a project can be relevant for other research contexts and in what way these research data can be made available to other researchers for subsequent use. In a proposal, applicants should therefore explain which research data will be created, generated or evaluated in the course of a scientific research project. This should be based on appropriate subject-specific concepts and considerations for quality assurance, for the handling and long-term storage of research data.”
- European Commission (EC)
The Commission is implementing a pilot project within the Horizon 2020 programme called the Open Research Data Pilot, which aims to improve access to and re-use of research data from Horizon 2020 projects. The Open Research Data Pilot follows the principle of “as open as possible, as closed as necessary”.
While only selected areas of Horizon 2020 were included in the 2014-2016 work programmes, the pilot now covers all subject areas in the revised version of the 2017 work programme.
The following regulations apply:
Creation of a data management plan according to the template. Submission within the first six months, updating in case of relevant changes or at least at the time of the intermediate and final evaluations of the project.
Data storage: Research data must be stored as soon as possible (applies to data underlying a project publication) or in accordance with the data management plan (applies to other data) in a suitable repository, preferably institutional, project-specific or subject-specific.
Publication: Where possible, the data should be published under an open licence (preferably CC-BY or CC-0) without any restrictions on use, including the required contextual information and tools.
However, a partial or complete exemption from the requirements on the basis of justified reasons is possible. (Does anything speak against publication?)
Further information can be found here:
Guidelines on FAIR Data Management in Horizon 2020 (PDF)
Guidelines on Open Access to Scientific Publications and Research Data in Horizon 2020
Horizon 2020 Online Manual: Open Access and Data Management (PDF)
Horizon 2020: Annotated Model Grant Agreement (AGA) (PDF)
OpenAIRE Research Data Management Briefing Paper (PDF)
- Publishers
Since publishers are also increasingly demanding the provision of the research data on which a publication is based, you should check the relevant requirements before publishing. We have compiled some examples for you below: |
Public Library of Science (PLoS): Data Availability / Materials and Software Sharing
Nature Publishing Group: Availability of Data, Materials, Code and Protocols
Science: Data and Materials Availability / Preparing Supplementary Materials
BioMed Central: Availability of Data and Material
Elsevier: Research Data Policy / Text and Data Mining Policy
Storing and Archiving Research Data
How do I structure my data in a useful way?
Since the work process often produces not only numerous data sets, but also respective versions due to various modification stages, it is advisable to make uniform specifications for file naming and versioning. This increases work efficiency and promotes collaborative work processes. It also enables long-term traceability and re-usability of data.
Furthermore, it may be useful to define separate folder structures for raw data, analysis data and data evaluations as well as other project materials. For more information on data organisation, see Jensen 2012, pp.40-42 (PDF).
Information on “File and Folder Organisation” is provided in this short lecture by Christian Krippes.
Read-only versions should be created at the various stages of modification (e.g., original data, cleaned data, data ready for analysis). Further editing should only be done in copies of these master files.
Due to the respective specifics of the research areas, but also of the data itself, naming conventions can be designed quite differently. However, they should always take into account the type of data files (original data, cleaned files, analysis files) and also the respective file form (working file, results file, etc.).
The save date should be included in the file name, follow the YYYYMMDD format and be placed at the beginning or end of the file name to facilitate sorting. Refrain from using special characters and symbols as well as spaces and use underscores instead. The names should always be consistent, clear and conclusive.
Examples of file naming are (see also HU Berlin: Structuring files):
- [Sediment]_[Sample]_[Instrument]_[YYYYMMDD].dat
[Experiment]_[Reagent]_[Instrument]_[YYYYMMDD].csv
[Experiment]_[Experimental Design]_[Subject]_[YYYYMMDD].sav
[Observation]_[Location]_[YYYYMMDD].mp4
[interviewee]_[interviewer]_[YYYYMMDD].mp3
The file names listed here follow the so-called “pothole case” (also “snake case”) writing style. This can be recognised by the underscores used as hyphens. Usually, the letters after each underscore are written in lower case, but capitalising all initial letters after the underscores is also possible.
In addition to the Pothole Case, there are also numerous other writing styles for naming files. One of the most common is the Camel Case. The following example shows a file naming in the Camel Case: “SedimentSampleInstrumentYYYYMMDD.dat”. Each word begins with a capital letter, there is no separation by an underscore, as in the pothole case, or any other special character. The disadvantage of this naming convention is, for example, the specification of versions (see next paragraph). A version 1.0.0 would be recognisable in the Pothole Case as 1_0_0, in the Camel Case as 100. Regardless of which style you choose, you should make sure to use the same one throughout the project.
Changes to the data can be indicated by specifying the version in the file name. A well-known concept of versioning based on the DDI standard (Data Documentation Initiative) is: Major.Minor.Revision.
Starting from version “1.0.0”, the following changes are made:
- the first digit if cases, variables, waves or sample have been added or deleted
- the second digit, if data are corrected so that the analysis is affected
- the third digit, when simple revisions without relevance to meaning are made
Versioning can also be supported by appropriate software (e.g. Git).
Which file formats should be used?
In order to be able to store data in the long term and use it sustainably, the choice of a suitable file format is of particular importance. As a general rule, make sure that files or formats are not encrypted, compressed, proprietary or patented. Accordingly, prefer open, documented standards.
Recommendations on which file formats should be preferred can be found at RADAR, HU Berlin, the UK Data Service or the Library of Congress, among others.
The following table also provides orientation:
Data type | Recommended formats | Less suitable or unsuitable formats |
---|---|---|
Audio | .wav / .flac | .mp3 |
Computer-aided Design (CAD) | .dwg / .dxf / .x3d / .x3db / .x3dv | - |
Databases | .sql / .xml | .accdb / .mdb |
Raster graphics & images | .tif (uncompressed) / .jp2 / .jpg2 / .png | .gif / .jpeg / .jpg / .psd |
Statistical data | .por | .sav (IBM®SPSS) |
Tables | .csv / .tsv / .tab | .xls / .xlsx / .xlx |
Texts | .odf / .rtf / .txt / PDF/A | .docx / .doc / PDF |
Vector graphics | .svg / .svgz | .cdr |
Video | .mov / .wmv |
Where do I save my data during the work process?
The regular backup of data in the work process is particularly important, considering possible technical and human errors. It is the responsibility of the researchers, who are supported in this by the university's infrastructure. Find out at your respective location what services are available.
What should I consider when backing up my data?
Good research data management is also characterised by the fact that you, as a researcher, are prepared as well as possible for a potential loss of data. For this reason, you should draw up a backup plan right at the beginning of your research project, which should ideally also include regular backup routines. The following questions should be answered in a backup plan:
- Which backup tool do you use?
- Of which data should a backup be created?
- Where should the backups of the data be stored?
- How often should backups of the data be made?
You should also regard the so-called 3-2-1 backup rule (see fig. 3). This means that you should always keep at least 3 copies of your data on 2 different data carriers (e.g., a USB stick and an external hard drive) and 1 at a decentralised storage location (e.g. the Hessenbox). It is important that all 3 copies are always up to date with the original file, which is why automated backup routines are best suited. Instructions on how to create automated backup routines with Windows Task Scheduling can be found here.
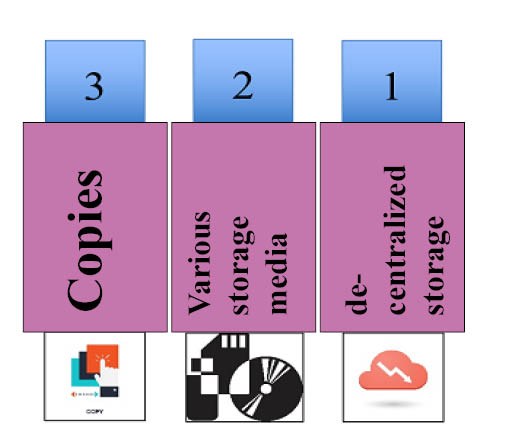
Fig. 3: 3-2-1 Backup rule
If you work with personal data or other legally sensitive data, please bear in mind that at least the backup at a decentralised storage location involves a backup on a tape that you no longer have any influence over. If you back up your data in the Hessenbox, for example, backups will be made in the facilities of the HRZ (IT service centre of the Justus Liebig University of Giessen). It is then difficult for you to comply with a possible request to delete the data. So please encrypt such legally sensitive data before storing it in a decentralised location. To do this, you can either create a zip folder which you password-protect, or you can use the tools VeraCrypt or Rohos MiniDrive. (What data protection restrictions must I observe?)
Where do I archive my data in the long term?
According to the principles of good scientific practice, research data should be stored for a period of at least 10 years. Subject-specific and interdisciplinary repositories are available for this purpose. (How do I find a suitable repository?)
Uploading data to a repository is not the same thing as publishing the data. For example, a period can be defined in which a data package is not yet accessible, but the metadata is already visible. Such embargo periods can be extended by a curator. Further information on the subject of “embargo” can be found here. In the case of publication, access and editing rights can also be regulated in contracts or through licences. (Can I control the use of my data? / Which licence should I choose? )
Uploading data to a repository is not the same thing as publishing the data. For example, a period can be defined in which a data package is not yet accessible, but the metadata is already visible. Such embargo periods can be extended by a curator. For further information on the subject of “embargo” please visit the linked site. In the case of publication, access and editing rights can also be regulated in contracts or through licences. (Can I control the use of my data? / Which licence should I choose? )
Please keep in mind the respective requirements of research funding and publishing houses as well as data protection regulations. (Who may decide on the dissemination and publication of data? / What data protection restrictions must I observe?)
Publish and Share Research Data
Why should I publish my data?
The publication of research data offers many advantages, not only for individual scientists, but also for the scientific community and the entire academic life.
On the one hand, published data sets can be quoted as an independent scientific achievement and increase the visibility of one's own research. This is because, as studies show, publications are quoted more frequently if the underlying data have also been published. (see Piwowar & Vision 2013)
On the other hand, data sharing enables the re-use of already existing data. In this way, new questions can be investigated, but at the same time duplication of work can be avoided and costs reduced.
Does anything speak against publication?
There are circumstances under which data shouldn’t be published, or such, where it should be published only under certain conditions. The most important prerequisite for publication is that you have the right to do so. (Who is allowed to decide on the transfer and publication of data? / Do I own the copyright to my data?)
On the other hand, it may be confidential personal data that may only be published after anonymisation or with the permission of the parties concerned. (What data protection restrictions must I observe?)
If you want to publish with a publisher, also make sure you choose the publisher wisely and do not fall for so-called predatory publishers. This short lecture by Werner Dees offers a brief overview of how you can recognise predatory publishers.
What data protection restrictions must I observe?
Personal data means “any information relating to an identified or identifiable natural person (person concerned); an identifiable natural person is one who can be identified, directly or indirectly, in particular by reference to an identifier such as a name, an identification number, location data, an online identifier or to one or more factors specific to the physical, physiological, genetic, mental, economic, cultural or social identity of that person” (Section 46 (1) BDSG, German Data Protection Act). They are subject to strict specifications in their collection, use and disclosure. For archiving, provision and publication, information that can be assigned to a specific or identifiable person should be removed from the research data. Depending on the data, diverse ways of anonymisation are suitable.
If personal data is to be processed, the consent of the data subject must usually be obtained. Among other things, the purpose must be clearly defined, and the party concerned must be able to assess the consequences.
In addition, research data such as company data may contain confidential information (know-how protection), or confidentiality and non-disclosure agreements may have been made that preclude publication.
Who may decide on sharing and publishing of data?
Possible owners or co-owners of the rights to the data are the researchers, the employers, the commissioning parties, research funding and/or (private sector) contractual partners. Who has a say in the dissemination or publication of research data, or who must be asked, is determined by the contractual relationship. Usually, the results of commissioned research belong to the employer or funder. The situation is different in the case of own research, where researchers are allowed to determine the data themselves.
Do I own the copyright to my data?
Research objects and occasionally also research data may be protected as works within the meaning of the Copyright Act. These may be linguistic works, computer programmes, musical works, pantomime works including dance works , works of fine arts including works of architecture and applied arts, photographic works, cinematographic works and representations of a scientific and technical nature.
Usually, research data lack the necessary level of creativity and are not works. It is possible, however, that certain types of research data are covered by ancillary copyright, for example photographs, moving images or sound recordings.
Often, the research data of a research project are protected by copyright as part of a database work or come under the ancillary copyright for databases.
Research data that are not covered by a property right can generally be used by anyone for any purpose without a licence or obligation to pay.
Can I control the use of my data?
If you have copyright or ancillary copyright over research data, you can regulate various aspects of use via appropriate contracts, such as the type and manner of use, user groups and time period, purpose, etc. Since contractual regulations for individual cases would be very time-consuming in practice, there are various solutions for standardised regulations of rights of use. For example, the Leibniz Centre for Psychological Information and Documentation (ZPID) offers standard contracts for the use of psychological data and GESIS regulates access restrictions for particularly sensitive social science data via user contracts. If you do not want your data to be subject to any specific access or use restrictions, the use of standardised licences such as Creative Commons or Open Data Commons is a good option. (Which licence should I choose?)
Which licence should I choose?
The publication of data under a specific licence allows a detailed definition of the permissible form of their use. This creates legal assurance on behalf of both the person providing as well as the one using the data. Even when waiving any restrictions, it is therefore important to formulate them.
Although data themselves are not usually subject to copyright, there is nevertheless something to be said for treating them as potentially worthy of protection, not least in order to express one's own ideas about further use. Various licensing models are available for this purpose. The most common of these is Creative Commons (CC). CC licences are independent of the licensed content and cover copyrights, ancillary copyrights and in the current version – if available – also database producer rights.
The Open Knowledge Foundation's ‚Open Data Commons' licence package has been designed specifically for the publication of data. In addition to the unconditional licence (Open Data Commons Public Domain Dedication and License (PDDL)), it offers three other models:
- Open Data Commons Attribution License (ODC BY v1.0): Condition of attribution
- Open Data Commons Open Database License (ODbL v1.0): Sharing under same conditions
- Database Contents License (DbCL v1.0): Sharing under the same conditions also for database contents
Regardless of its legally binding nature, the CC-BY licence certainly comes closest to fulfilling the idea of Open Access and Open Science, whereas the 'distribution under the same conditions' can lead to compatibility problems with other licences and the prohibition of editing can lead to restrictions in use by data mining for example or also to problems with long-term archiving. Prohibiting commercial use hampers use in commercial databases and thus potentially reduces the visibility of your research.
Whichever licence you choose, you should take the decision in a deliberate and informed way. A more detailed discussion of the topic can be found in Wiebe & Guibault 2013. This presentation by Frank Waldschmidt-Dietz also provides a brief overview of Creative Commons licences and licensing in general. Frank Waldschmidt-Dietz as well shows the advantages of free, open access licences for education in a video on Open Educational Resources, or OER for short.
Regardless of the terms of use, the rules of good scientific practice apply, of course, which require that the source of data used be stated.
How can I publish data?
Both interdisciplinary and discipline-specific repositories are available for publishing data. The latter offer various advantages and should therefore be preferred: To begin with, subject-specific standards and metadata schemas are better accounted for here. In addition, more specific indexing and search options improve the findability of the data. If they are stored in a subject-specific repository, this also increases visibility within the subject community. You can find the subject-specific repositories relevant to you at the Consortia of the National Research Data Infrastructure.
Interdisciplinary repositories include the EU-funded Zenodo, Dryad and Figshare.
In addition, data can also be published in data supplements of journals. Although this form of data publication is becoming increasingly important, additional archiving options should be used to ensure long-term availability.
How do I find a suitable repository?
If you are looking for a suitable repository, answering the following questions may be helpful:
-
Is the repository appropriate for the subject?
-
Is the repository certified (e.g. CoreTrustSeal or/and FAIR-compliant)?
-
Is it established and linked to specific search portals?
-
Does the repository offer the desired services (e.g. persistent identifiers, open access, differentiated access rights, implementation of embargo-periods)?
-
Is the repository guaranteed to be sustainable?
-
Is there an exit strategy or an agreement to preserve the data if funding ceases?
-
How are data transfer and data use regulated in terms of content and form?
To find a suitable repository, you can use the Registry of Research Data Repositories (re3data.org). This is a web-based directory in which research data repositories are indexed. The search for a suitable repository can be carried out as a simple search. Numerous filters also allow you to narrow down the search, e.g., by subject area or data type. You can access the search here.
What must I consider when feeding data into a repository?
- Format
First of all, it is important that the data is available in a suitable format. Some repositories have stricter specifications here, others merely make recommendations or are open to all formats. This makes it all the more important to think about this in advance of the research. (How do I create a data management plan?) For general advice and specific links on formats, see: Which file formats should be used?
- Metadata
In order for data to be found and used well, it must be documented precisely by metadata. Please have a look at the detailed notes at: What are metadata, metadata schemas, controlled vocabularies and documentation?
- Publication
An upload to a repository does not automatically mean immediate publication. Under certain circumstances, there may be reasons for an embargo period or partial publication. Especially in business-related research disciplines, embargoes on research results are common. Therefore, consider whether there are important reasons against immediate publication. (Does anything speak against publication?)
- Conditions
Also consider the conditions under which you want to publish your data. There are various licence models for this (Which licence should I choose?).
What are metadata, metadata schemas, controlled vocabularies and documentation?
Metadata are data on other data or resources, in this case research data. They describe the research data in order to optimise their retrievability, to ensure the understanding of the data for subsequent users and also enable the linking of similar research data when using the same, standardised metadata schemes. The most basic metadata information includes, for example, title, author/primary researcher, institution, persistent identifier, location & time period, subject, rights, file names, formats, etc.
Metadata schemas (or metadata standards) are compilations of categories for describing data. A distinction is made between interdisciplinary or independent standards and discipline-specific or dependent standards. Metadata schemas are intended to ensure that all researchers use the same descriptive vocabulary in order to guarantee interoperability and thus comparability of data sets.
The following table lists some examples of metadata standards from different disciplines. If your discipline is not listed, the listing of the Digital Curation Centres (DCC) can usually provide information on which standards are applicable to your field of science.
Scientific or technical discipline | Name of the standard(s) |
---|---|
interdisciplinary standards | DataCite Schema, Dublin Core, MARC21, RADAR |
Humanities | EAD, TEI P5, TEI Lex-0 |
Earth Sciences | AgMES, CSDGM, ISO 19115 |
Climate science | CF Conventions |
Arts & Cultural Studies | CDWA, MIDAS-Heritage |
Natural sciences | CIF, CSMD, Darwin Core, EML, ICAT Schema |
X-ray, neutron and muon research | NeXus |
Social and economic sciences | DDI |
Before starting to document your data, you should search for existing metadata schemas. This approach ensures better interoperability of the research data you are creating with data already created in the same discipline and saves you the work of developing your own metadata schema. If an existing metadata standard does not provide the descriptive categories that are necessary for your research, it is still worthwhile to use a reputable, already existing subject-specific standard as a basis and build on it, for example by incorporating additional categories and communicating this to those responsible for the standard so that they can extend the schema. This is because metadata standards are living entities that can be adapted or enriched with new categories depending on the needs of the researchers. Be careful not to make any changes to existing elements or attributes so as not to jeopardise interoperability.
It is possible and usually necessary to use several metadata schemas. At the very least, you should always use a subject-independent metadata schema (preferably Dublin Core) to describe your data, as this covers the general categories of description mentioned in the first paragraph of this section about the research data created. Fig. 4 shows a section for metadata in the Dublin Core standard for illustration purposes. Subject-specific metadata standards, on the other hand, allow you to structure your data with descriptive categories that are on a more content-related level and may differ from discipline to discipline.
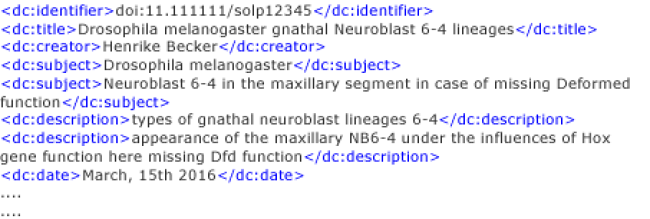
Fig. 4: Example metadata in the Dublin Core Standard
Type of controlled vocabulary | Name of the controlled vocabulary |
---|---|
Clear identification of persons, Objects or places |
Gemeinsame Normdatei (GND) GeoNames / International Standard Name Identifier (ISNI, ISO 27729) / |
General, interdisciplinary Classification systems |
Dewey-Dezimalklassifikation (DDC) / |
Subject-specific classification systems | |
Subject-specific vocabularies |
Agricultural Information Management Standard (AGROVOC) Standard-Thesaurus-Wirtschaft (STW) Thesaurus Sozialwissenschaften (TheSoz) |
Metadata schemas thus determine what information is to be provided. For optimal search and use of the data, however, it is also important that this information is rendered using a vocabulary that is as uniform as possible. For this purpose, a number of discipline-specific and cross-disciplinary controlled vocabularies are available in the form of thesauri, classifications and standard data.
An overview of different systems is provided, for example, by the Basel Register of Thesauri, Ontologies & Classifications (BARTOC).
Documentation usually goes beyond the description of the data via metadata. It represents a deeper (scientific) indexing, in the framework of which, for example, the context of origin, variables, instruments, methods etc. are described in detail and thus the provenance of the data becomes apparent. In many cases, such a description is indispensable in order to understand, verify and, if necessary, use the data.
Introductions to the topic of metadata are offered, for example, by the JISC Guide or the interactive Mantra course of the University of Edinburgh.
What are Persistent Identifiers?
Persistent identifiers (PID) uniquely reference a digital resource via a code. This code is a unique, unchangeable designation that can be used to link permanently to this resource. The unique referencing makes the linked content citable. (How do I cite research data?) It does not matter whether it is a research dataset, journal article, video or other resource. The persistent identifier thus ensures that the resource can still be retrieved even if, for example, the internet address of the server changes. Persistent identifiers thus play a key role in preserving research data and making them available in the long term.
There are different forms of PIDs. An increasingly common form is the Digital Object Identifier (DOI). This DOI is assigned only once worldwide. If you put “https://www.doi.org/” in front of the DOI, it links to the article from o-bib.de (see https://www.doi.org/10.5282/o-bib/2018H2S14-27). In addition to the DOI, the use of a Uniform Resource Name (URN) as a unique identifier is also common for online publications, for example.
The relevant publication platforms for research data such as Zenodo and Figshare automatically reserve a DOI when you publish your data, which this data set then receives. If you publish in another, subject-specific repository, you should make sure that it also offers DOIs or another form of PIDs. (How can I publish data? / How do I find a suitable repository?)
Find and Use Research Data
Where can I find research data?
Not least because of the requirements and recommendations of funders, publishers and institutions for making data accessible, research data are increasingly available for subsequent use. In order to find suitable research data for your research area, relevant offers from your field are often the first port of call. These can be institutional or subject-specific repositories or data journals. You can find repositories – broken down by subject – at re3data.
In addition, it is also possible to search for data via generic search services. A major disadvantage of these search services is that they often cannot adequately map the detailed metadata schemas of their sources. Furthermore, the respective metadata differ greatly in terms of what they identify, i.e., individual data, data sets or collections.
Three well-known search services are
Retrieves metadata from repositories and databases via OAI-PMH. Research data can be found via the document type “Primary data”.
Searches metadata from various sources such as CLARIN or Global GBIF.
Searches metadata of information objects, including research data (object type 'dataset'), which are registered with DOIs at DataCite. Some of the metadata are also queried by the other two services.
For the subsequent use itself, the respective rights (licences, user contracts, if applicable) are binding. Among other things, they can determine who may use the data for what purpose and for how long.
If you do not want to or cannot use existing research data, you can, of course, use suitable research methods from your discipline to obtain data. The Coffee Lecture by Dr Samuel de Haas and Jan Thomas Schäfer on the YouTube channel of the JLU Giessen University Library, for example, offers information on data extraction via web scraping and text mining and on dealing with Big Data in practice.
How do I cite research data?
In order to adequately document the use and subsequent use of one's own and other people's research data in the sense of good scientific practice, correct data citation is essential.
In the case of external data, this also acknowledges the scientific achievement of its 'originator'. As with the citation of other publications, the conventions for citing data may differ formally. In terms of content, however, they are united by the requirement of unambiguous identifiability of the data source. The FORCE11 Data Citation Synthesis Group has developed recommendations for data citation. According to them, a complete data citation includes:
Author(s), year, title of research data, data repository or archive, version, worldwide Persistent identifier
Other optional information that can be useful in the context of a citation is Edition, URI, Resource Type, Publisher, Unique Numeric Fingerprint (UNF) and Location (cf. Alex Ball & Monica Duke 2015) (PDF).